Complete Guide to Random Bin Picking
What is Random Bin Picking?
Random bin picking is a robotic automation task in which a robot, aided by a 3D camera, picks objects that are arranged randomly, with unknown orientations and unstructured positions, from a bin or container. This process is typically implemented in manufacturing and warehouse environments, with the primary goal of automating the retrieval of items from a random or unstructured pile.
Random Bin Picking Industries and Examples
Random bin picking is invaluable in industries where handling and sorting objects from bins or containers is repetitive, labor-intensive, and time-consuming. Below are several examples of bin picking applications across various industries.
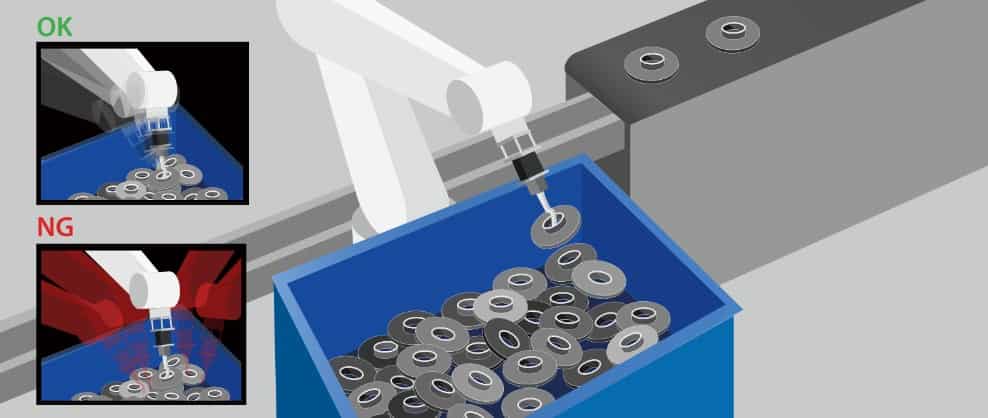
Random Bin Picking of Metal Objects
Logistics
In logistics and warehousing, random bin picking is used to sort and handle numerous packages positioned randomly within bins. For instance, in a project for a global e-commerce platform, assorted packages were picked from bins and placed on a conveyor belt at high speed. The primary challenge was maintaining rapid picking rates while safely handling heavy packages to avoid handling errors or damage. Solomon’s AI solution enabled the system to operate swiftly, meeting high throughput demands while ensuring accurate and safe handling of varying package sizes and weights.
Automotive
In the automotive industry, random bin picking is utilized to handle and sort automobile components. The main challenge for bin picking systems is achieving high accuracy to ensure each part is correctly classified, picked, and properly stacked. In a project for a major Japanese car manufacturer, AccuPick was employed to precisely handle auto parts, avoiding errors and defects in assembly and placement, thereby improving overall efficiency.
Food and Beverage
In the food industry, random bin picking plays a crucial role in automating the sorting and handling of food products, significantly enhancing operational efficiency. One of Solomon’s notable projects in this sector involved collaboration with a leading multinational confectionery company to pick packaged cookies from bins and transfer them onto a conveyor belt. The primary challenge was cycle time, as the system needed to pick and place approximately 30 to 35 cookies per minute. Automation was implemented to reduce the physical strain on operators, thereby minimizing the risk of musculoskeletal injuries, ultimately enhancing both workplace safety and production line efficiency.
Manufacturing
Bin picking is widely used in manufacturing to pick and sort various components. A leading producer of industrial robots and automation machinery required high-speed random bin picking of specific metal items. The main challenges were stringent cycle time and accuracy requirements. The objects needed to be stacked at the drop station, and any error in picking accuracy would result in incorrect stacking. The items could be positioned from either a top or back view, with varying inclination angles, requiring AI to accurately detect each side and its angle for precise placement. The shiny surface of the metal items posed an additional challenge for 3D cameras due to light reflection, which could distort both the 2D image and 3D point cloud, reducing picking accuracy. To overcome this, a SolScan industrial 3D camera equipped with a green projector light was used instead of the usual white light.
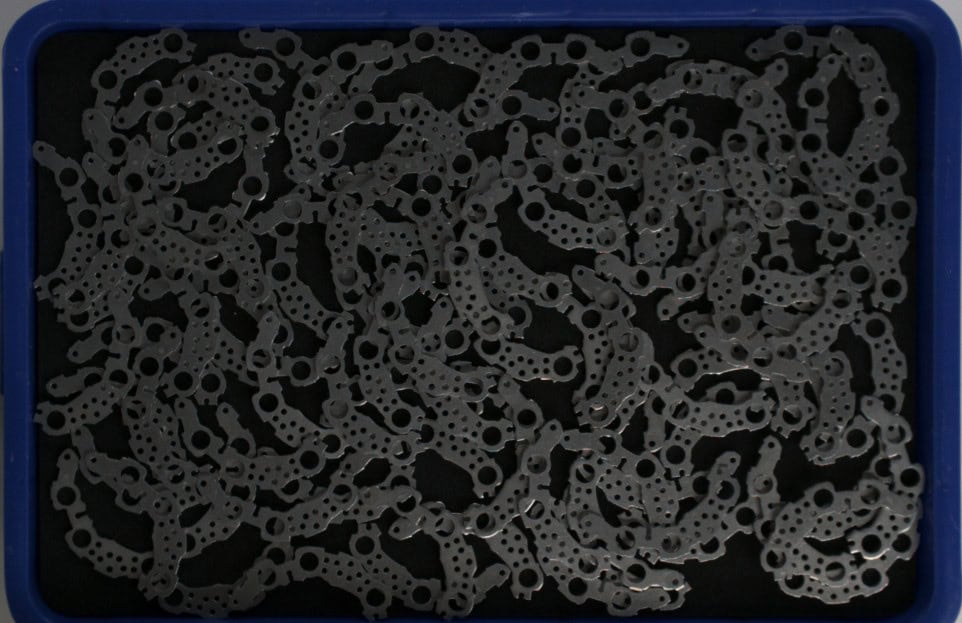
Original 2D Image
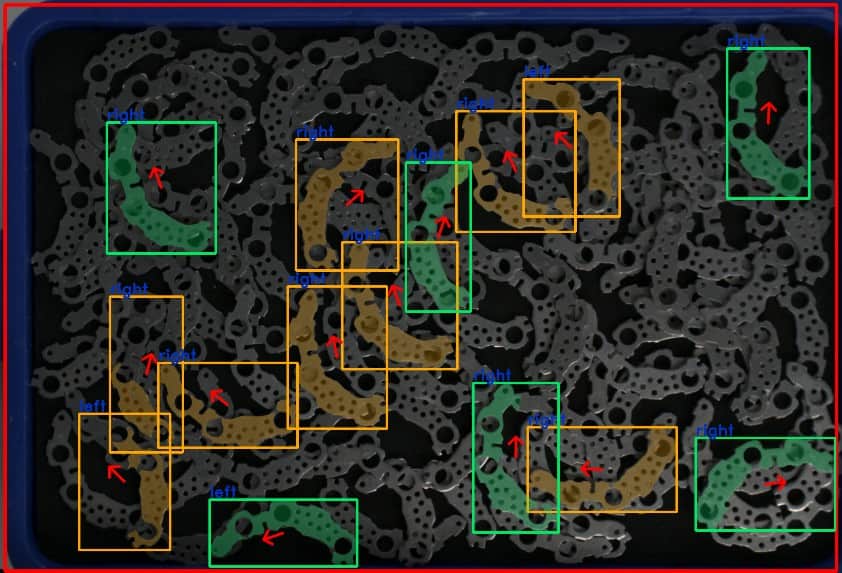
2D AI Detection with Angle Recognition
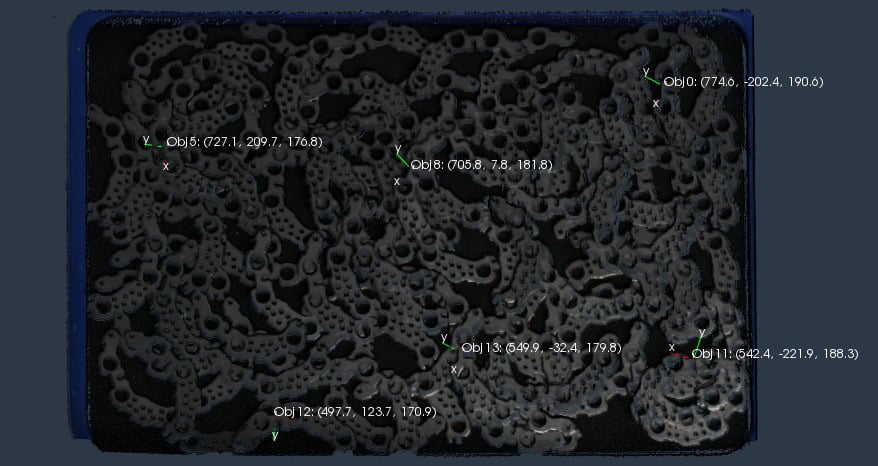
3D Point Cloud with Pick-Point Placement
Pharmaceuticals and Medical
In the pharmaceuticals and medical industry, bin picking systems are essential for handling diverse items in high-speed environments where precision is critical. In this case, a pharmaceutical distributor managed various containers, including boxes and bottles of different shapes, sizes, and colors, with approximately five objects in random positions and orientations per bin. The primary challenge was ensuring the product’s barcode remained visible during picking, as the items needed to be positioned between barcode readers for scanning. The working area was limited, and robots had to operate at high speeds to meet strict cycle times, increasing the risk of collisions. AccuPick was used to address this. Its motion planning software calculated safe, collision-free trajectories, while built-in AI enabled high-accuracy detection, allowing the robot to pick objects from any position (top, side, or bottom) without occluding the barcode. Two distinct robot end-effectors—one with a large suction cup and another with a small suction cup—were employed, and based on object detection results, position, and type (box or bottle), strategic conditions and rules were established to ensure the appropriate end-effector was selected for each specific object case.
Random Bin Picking System Essentials
Tool Center Point (TCP) Calibration
The TCP calibration of the robot must be performed once an end-effector is selected for the application and is mounted on the robot’s flange. The TCP serves as the reference point for instructing the robot on the precise position and orientation of the end-effector.
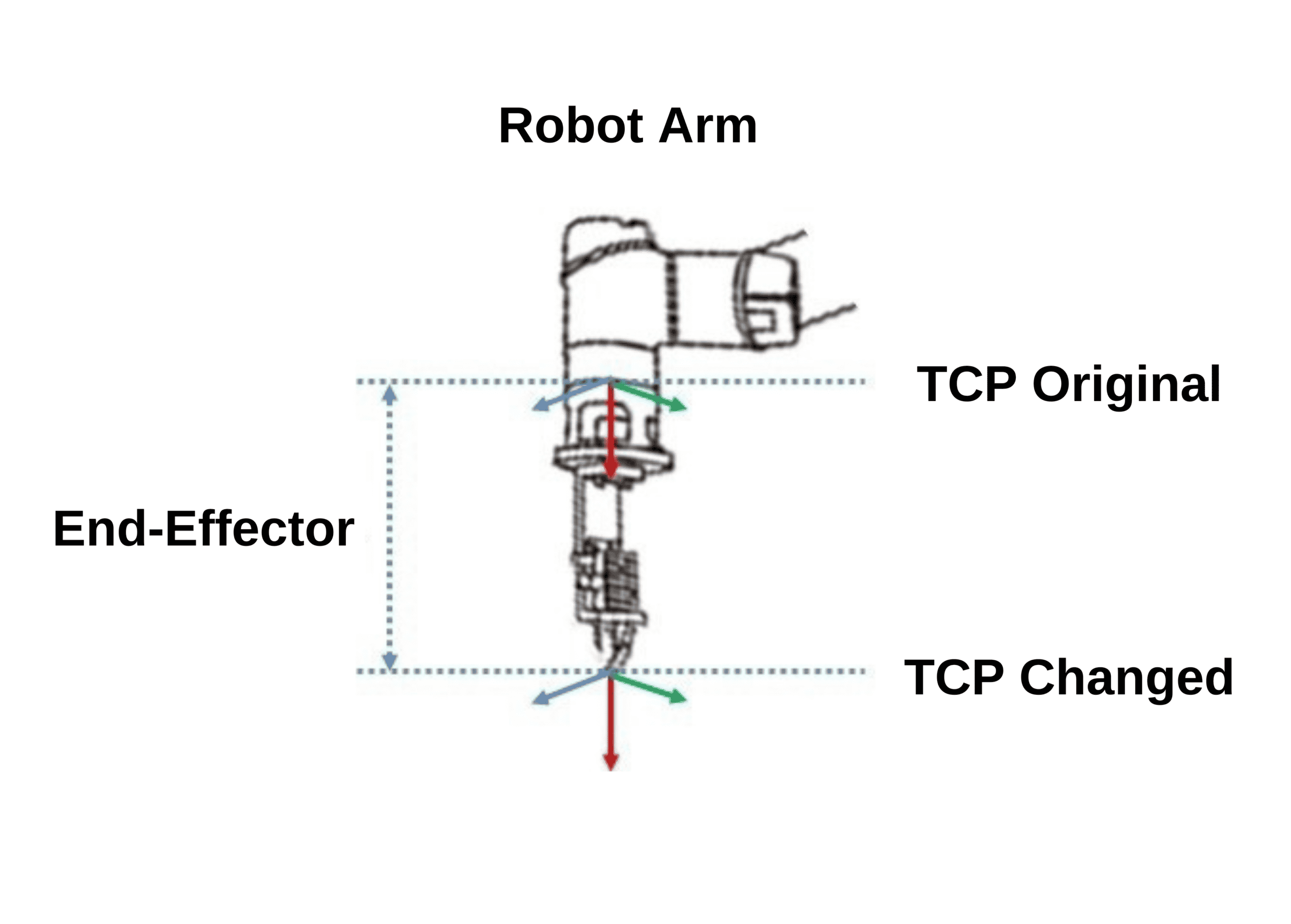
TCP Calibration
Vision to Robot Calibration
Calibration between the vision system and the robot is essential. As the 3D camera has its coordinate origin located at the base of the camera and the robot has its coordinate origin at the base of the robot, calibration allows the robot to understand the camera’s coordinate system and convert these coordinates to its own. As a result, any positional and rotational coordinates sent from the 3D scanner to the robot will be accurately interpreted, enabling the robot to reach the specific point with the required position and rotation.
3D Scanning and Object Detection
The 3D scanner captures both 2D images and 3D point clouds of the objects in the bin. This data is used to generate a pick-point for objects that meet the basic criteria for picking. These criteria can vary by project but generally include:
• Objects located on the top surface of the bin.
• Objects that are not occluded or are only slightly occluded by other items.
• Visible features that aid in determining the picking orientation of the object.
• Objects are positioned and rotated safely to ensure the robot or its end-effector will not collide during picking.
• Objects located on the top surface of the bin.
• Objects that are not occluded or are only slightly occluded by other items.
• Visible features that aid in determining the picking orientation of the object.
• Objects are positioned and rotated safely to ensure the robot or its end-effector will not collide during picking.
Pick-Point Generation and Execution
The pick-point for each object typically includes both positional and rotational coordinates. Once generated, the robot receives this pick-point. Then, the robot controller calculates the trajectory to reach it. For more challenging applications in environments that require collision avoidance, motion planning software is necessary to calculate a safe trajectory. Upon reaching the pick-point, the robot picks the object and then drops it in the desired location or bin.
Cycle Repetition
Once all detected objects in the current cycle have been picked and dropped, a new cycle begins from the scanning step. This ensures continuous operation until the bin is empty.
Random Bin Picking Challenges and Solutions
Strict Cycle Time Requirements
Projects with strict cycle time requirements face significant challenges, as each step must be completed swiftly to meet overall goals. Key considerations include:
• 3D Camera Capture Time: The 3D camera must quickly capture both 2D images and 3D point clouds.
• Image and Point Cloud Generation: Algorithms to generate the 2D images and 3D point cloud must be efficient and optimized.
• Object Identification: The object identification algorithm needs to be fast; leveraging AI can greatly enhance speed and accuracy.
• Pick-Point Placement: The algorithm for determining pick-points must be rapid. AI can be used here to achieve high speed.
• Motion Planning: The motion planning system must swiftly calculate safe trajectories.
• Robot Speed: The robot itself needs to operate at high speeds to meet cycle time demands.
• End-Effector Selection: Choosing the appropriate end-effector is crucial for minimizing failed picks and ensuring objects remain secure during robotic movements.
• 3D Camera Capture Time: The 3D camera must quickly capture both 2D images and 3D point clouds.
• Image and Point Cloud Generation: Algorithms to generate the 2D images and 3D point cloud must be efficient and optimized.
• Object Identification: The object identification algorithm needs to be fast; leveraging AI can greatly enhance speed and accuracy.
• Pick-Point Placement: The algorithm for determining pick-points must be rapid. AI can be used here to achieve high speed.
• Motion Planning: The motion planning system must swiftly calculate safe trajectories.
• Robot Speed: The robot itself needs to operate at high speeds to meet cycle time demands.
• End-Effector Selection: Choosing the appropriate end-effector is crucial for minimizing failed picks and ensuring objects remain secure during robotic movements.
High Picking Accuracy
Achieving high picking accuracy is vital for effective random bin picking. Key considerations include:
• Appropriate 3D Scanner: Selecting a 3D scanner that is suitable for the object material, working distance, and bin dimensions is essential.
• High Resolution: The 3D scanner should feature high resolution for detailed capture.
• Computer Vision and Image Preprocessing: Utilizing advanced computer vision techniques and image preprocessing can significantly enhance accuracy.
• AI for Object Detection: Selecting the correct AI algorithm can improve object detection accuracy.
• AI for Pick-Point Placement: AI 3D matching methods can further refine the accuracy of pick-point placement.
• Fine-Tuning: Comprehensive fine-tuning of the 3D scanner parameters, image preprocessing techniques, 2D detection AI algorithm, and 3D matching method for pick-point placement is essential for optimal performance.
• Stable Lighting Environment: Ensuring a stable lighting environment is important for achieving consistent results.
• Appropriate 3D Scanner: Selecting a 3D scanner that is suitable for the object material, working distance, and bin dimensions is essential.
• High Resolution: The 3D scanner should feature high resolution for detailed capture.
• Computer Vision and Image Preprocessing: Utilizing advanced computer vision techniques and image preprocessing can significantly enhance accuracy.
• AI for Object Detection: Selecting the correct AI algorithm can improve object detection accuracy.
• AI for Pick-Point Placement: AI 3D matching methods can further refine the accuracy of pick-point placement.
• Fine-Tuning: Comprehensive fine-tuning of the 3D scanner parameters, image preprocessing techniques, 2D detection AI algorithm, and 3D matching method for pick-point placement is essential for optimal performance.
• Stable Lighting Environment: Ensuring a stable lighting environment is important for achieving consistent results.
Random Object Orientation
Objects in bins often have random arrangements, unknown orientations, and unstructured positions. This randomness can cause objects to impede each other. To overcome these challenges, several strategies can be employed:
• Layer-by-Layer Approach: Scan and pick the most suitable objects, then rescan to initiate the next iteration, proceeding layer by layer.
• Bin Shaker: Use a bin shaker to alter the positions of the objects.
• Two-Stage Picking: First, pick the object as effectively as possible, and then separate it at a secondary station without other objects for more accurate retrieval.
• End-Effector: The correct selection of the end-effector is crucial for increasing the likelihood of successfully picking objects in challenging scenarios.
• Bin Design: The design of the bin is pivotal. For certain applications, an inverted truncated pyramid bin shape may offer an effective solution.
• Layer-by-Layer Approach: Scan and pick the most suitable objects, then rescan to initiate the next iteration, proceeding layer by layer.
• Bin Shaker: Use a bin shaker to alter the positions of the objects.
• Two-Stage Picking: First, pick the object as effectively as possible, and then separate it at a secondary station without other objects for more accurate retrieval.
• End-Effector: The correct selection of the end-effector is crucial for increasing the likelihood of successfully picking objects in challenging scenarios.
• Bin Design: The design of the bin is pivotal. For certain applications, an inverted truncated pyramid bin shape may offer an effective solution.
Incomplete Picking Accuracy
Despite the capabilities of advanced vision systems, picking accuracy may not always be 100%. Objects positioned at the bottom, edges, or corners of the bin may remain unpicked due to their location. In these instances, employing a bin shaker to reposition the objects to more accessible areas within the bin can be an effective solution.
Limited Working Space and High Robot Speed
In confined spaces where high-speed robot movement is necessary, motion planning software is essential. A lightweight CAD file of the workspace and end-effector may be required, which should include the robot model to simulate potential trajectories and calculate the safest path.
Transparent or Shiny Objects
Transparent or shiny metal objects present additional challenges in generating an accurate point cloud for pick-point placement. Solutions include employing AI, computer vision, and image preprocessing techniques, as well as utilizing a high-quality 3D scanner. Combining methods such as stereo vision and structured projector light, along with options for different light colors, high resolution, and increased light intensity, can effectively help to address these challenges.
Random Bin Picking Using AI
AI is instrumental in achieving high-quality detection and precise pick-point placement while maintaining competitive cycle times in industrial applications. When combined with computer vision and image preprocessing techniques, AI can adapt to various environmental conditions and object orientations. This adaptability makes AI especially suitable for random bin picking applications, where objects are randomly arranged. AI models developed for these scenarios should be robust and flexible, capable of handling diverse picking challenges.
Random bin picking, powered by AI and advanced vision systems, is transforming industrial automation across various sectors. Explore how Solomon’s AI and 3D vision solutions can optimize your operations.