What Are the Differences Between AI and AOI?
The transition from an era of manufacturing characterized by mass production with limited variety to today’s dynamic model emphasizing the production of low-volume, high-variety goods has made quality control and inspection more complex and challenging. Goods now come in a wider range of shapes, sizes, materials, and other features, often leading to more undefined defects.
Consequently, AOI (Automatic Optical Inspection) systems have become the prevailing solution for defect detection in industrial processes. Yet conventional AOI depends on predefined defect samples and fixed parameters for defect detection, restricting the capacity of AOI systems for agile and swift inspection. The likelihood of traditional inspection systems missing defects increases substantially; however, AI offers a transformative solution for machine vision by facilitating more flexible and efficient inspections.
Defect Detection Using AOI
As consumer electronics products continue to advance, industries such as semiconductors, optoelectronics, and smart manufacturing are experiencing substantial growth. With defects capable of impacting product functionality, there’s a growing need for enhanced yield in manufacturing sectors. Traditional production lines have relied on manual inspection of product defect patterns, but this method fails to meet the demand for thorough inspection and production efficiency.
AOI systems, powered by machine vision technology, seamlessly combine optics, electronic control, mechanics, and inspection software to perform precise inspection tasks. These systems improve defect detection efficiency by highlighting image features through optimized light source configurations and utilizing advanced computer image processing techniques for accurate positioning and inspection. By quickly screening for defects, contaminants, and irregularities in both finished and semi-finished products on the production line, AOI can greatly enhance quality control measures.
Compared to manual inspection methods, AOI systems provide a superior level of consistency in defect detection. Moreover, AOI systems equipped with high-resolution cameras can discern intricate details that may be difficult for the human eye to detect. Additionally, their capacity to inspect thousands of items per minute addresses the inefficiencies inherent in traditional manual visual inspection processes.
AOI Industrial Applications
AOI technology is widely used across various industrial sectors to inspect products for deformities, damage, missing components, dirt, scratches, and other defects, while also performing critical tasks such as barcode reading, precise measurements, and printing inspection. As a result, various industries including electronics, textiles, food and beverage, and automotive benefit from AOI technology.
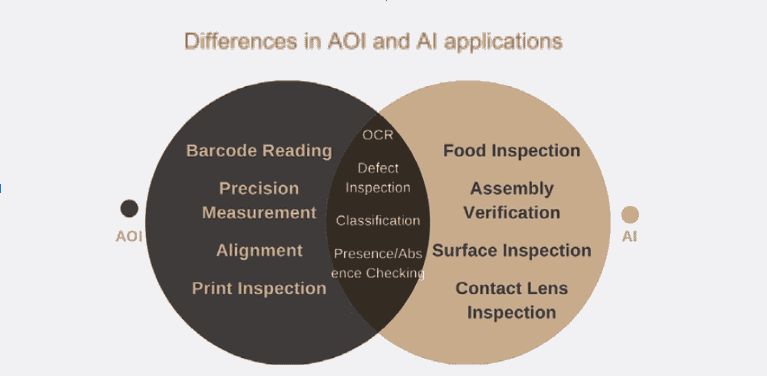
Limitations of AOI for Defect Detection
While AOI adoption has become commonplace across various industrial sectors, the highly customized nature of AOI presents challenges and limitations to widespread implementation. Particularly when faced with complex product defects, image backgrounds, and variations in lighting, AOI systems may encounter constraints.
AOI operates on a rule-based judgment mechanism where detection logic is programmed into the system. AOI can only identify defects based on predefined parameters established as the benchmark for inspecting sample defects. For instance, if the detection logic defines defects as circular, any non-circular defects may go undetected by the AOI system. Randomly generated defect types, such as variations in eggshell quality, abnormal defects in PCBA assembly, oil stains on plastic buckles, and scratches on metal stamped parts, pose significant challenges for AOI to detect such complexities effectively.
To mitigate the risk of missed defects, AOI systems are often set to parameters with a high level of strictness, making AOI susceptible to false positives. Moreover, system operators must continuously fine-tune the system algorithms and parameters, while production lines may require repeated product inspections to prevent the oversight of defects or false positives. These factors significantly impact production efficiency and drive up production costs.
The effectiveness of AOI depends on the precise coordination of light sources, cameras, and lenses. Regarding light sources, adjusting their intensity and characteristics is crucial to enhance contrast and effectively display object features. This adjustment must be customized to the material, color, and shape of the object under inspection to obtain optimal images and reduce the risk of false positives. When selecting cameras, factors such as sensitivity components, resolution, and frame rates must be carefully considered based on inspection requirements. These cameras must be paired with suitable lenses to fully exploit the capabilities of the optical system.
Aside from the complexities of equipment details affecting imaging, operators must also handle the task of reconfiguring machine parameters and alignment when adjustments or component replacements are needed, drawing on their experience. Moreover, adjusting hardware and software components is inherently complex, and repair times can vary widely, ranging from a few days to several months. This presents challenges in meeting the dynamic demands of modern production lines. As a result, many industries that utilize AOI have recently embraced artificial intelligence (AI) to improve inspection accuracy and efficiency.
To delve deeper into the advantages of integrating AI with AOI, click here.
Defect Detection Using AI
The main difference between AI-based inspection and traditional AOI defect detection lies in their reliance on empirical rules. AI operates like a new apprentice, capable of mimicking the inspection abilities of a master craftsman to some extent. AI can autonomously define defect ranges and abnormal detection models by utilizing large amounts of valid data and training the system model using deep learning techniques. AI can automatically identify optimal parameters for both acceptable (OK) and non-acceptable (NG) defects. This enables effective detection of unknown defects, ensures consistency in inspection standards, and greatly enhances inspection accuracy.
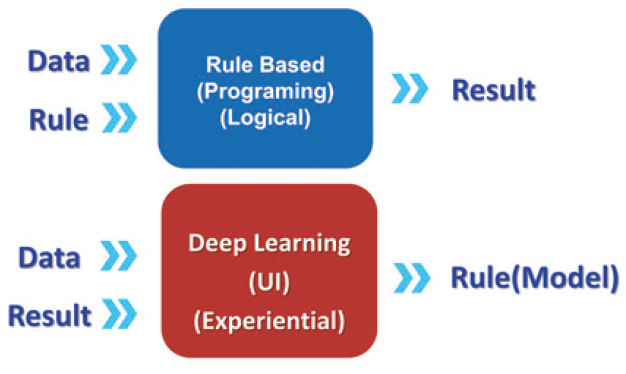
Due to significant variations in domain knowledge across industries, traditional AOI engineers developing AOI systems for specific sectors may face challenges in promptly addressing practical field issues. Therefore, effective solutions often require collaboration with industry experts to design suitable AOI hardware and rules tailored to the industry’s needs. In contrast, AI-based defect detection systems learn the required image features for detection through interfaces. Once trained, AI systems can autonomously generate detection rules. There are notable differences in learning efficiency and execution power between these two approaches.
In addition to optimizing detection accuracy through deep learning, AI technology notably addresses issues related to the impact of light sources on detection performance. Traditional AOI systems often face limitations due to lighting conditions, imaging angles, shadow reflections, and the material and shape of the object under inspection (especially semi-transparent materials and metallic surfaces). These limitations frequently impede the achievement of accurate detection results. In contrast, the accuracy of AI-based inspection is less dependent on light sources. With a stable light source, AI can effectively perform defect detection and classification even in scenarios with blurred contours, different shapes, and interference from reflective metallic materials.
AI defect detection excels at intricate surface and appearance defects, such as dents and scratches on reflective objects. Moreover, even when inspecting deformed or less recognizable characters, AI can be proficient at OCR (Optical Character Recognition) tasks. Additionally, AI-based systems are adept at screening unpredictable patterns and defects, regardless of variations in color and size, extensive deformations, complex shapes, or random scattering, AI comprehensively analyzes data, accurately determining the presence of defects.
AI vision systems therefore outperform traditional rule-based AOI systems in terms of speed, precision, and labor costs, addressing the challenges inherent in complex detection and classification applications.
Defect Detection Using AI Case Study
In the pharmaceutical industry, blister pack production lines typically handle between 5,000 and 40,000 pills per hour. However, errors can occur during filling, resulting in defects like empty blisters, low-quality contents, or powder spillage from capsules. By training AI models in SolVision with a variety of defect images, each pack and filling condition can be quickly and accurately identified, labeled, and classified.
Learn more about blister pack inspection using AI in this case study.