SolVisionPetrochemicals, Plastics, and Rubber
Case Study
Injection Molded Rubber Inspection Using AI
Case
Injection Molded Rubber Inspection
The injection molded rubber process is influenced by various factors, including raw material quality, machine specifications, mold design, and injection parameters. Potential defects can arise, ranging from minor aesthetic issues like stains and scratches to severe structural damage caused by inadequate materials or mishandling during ejection. Effective rubber inspection is crucial for identifying these defects, ensuring quality control, and optimizing production processes with advanced solutions like AI.
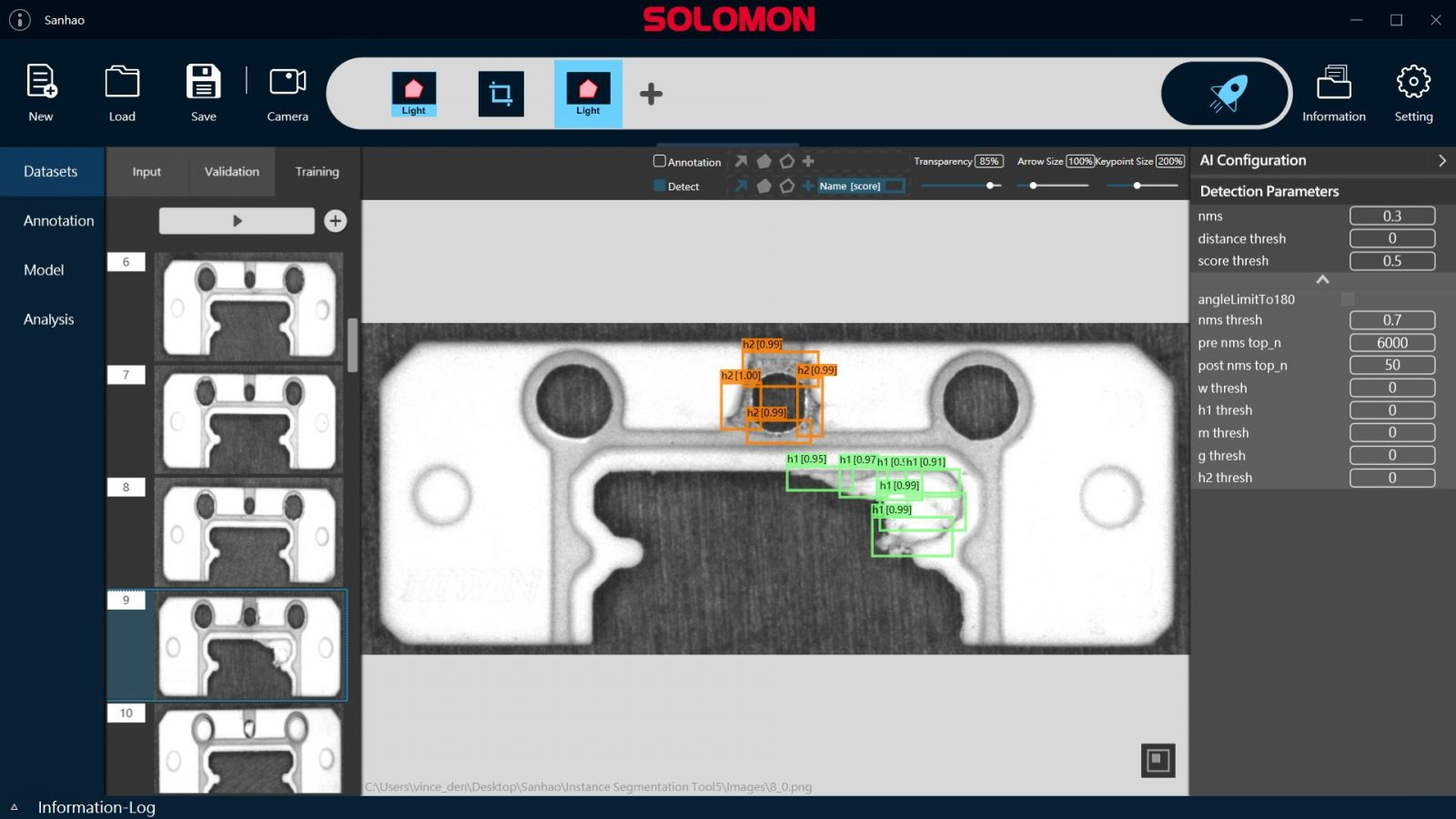
Challenge
Limitations of Traditional Inspection Methods
Conventional rule-based vision systems rely on extensive training data. However, they struggle with the dynamic nature and varying locations of defects in injection molded rubber, often resulting in low accuracy rates. Furthermore, manual inspection lacks standardization and is frequently too slow and inconsistent for effective quality control. These challenges underscore the need for advanced AI solutions to enhance rubber inspection processes.
Solution
Precise Quality Inspection with SolVision
SolVision AI employs deep learning to inspect injection molded rubber by analyzing sample images to learn the distinct characteristics of various defects, enabling precise defect detection and identification. As the database is enriched with additional images, the AI model improves, further enhancing the system’s accuracy in defect recognition. This advancement streamlines the rubber inspection process, providing manufacturers with faster, more reliable quality control.
Injection Molded Rubber Defect Detection
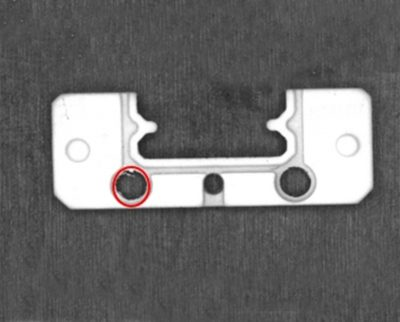
Uneven Incisions
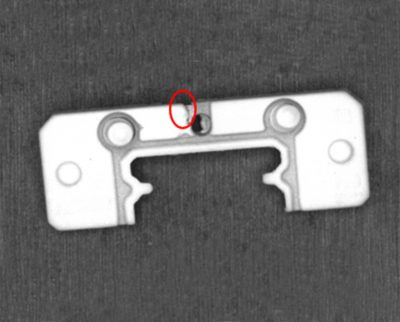
Missing Material
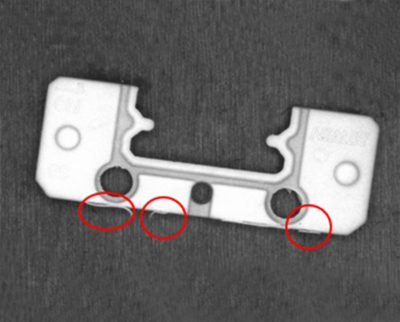
Mold Crush
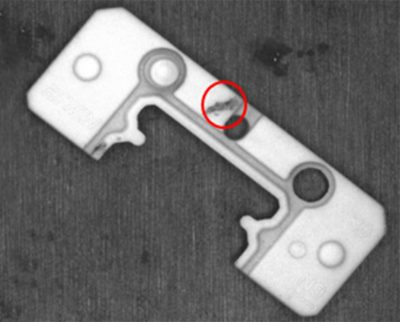
Stain