META-aiviCase Study
AI Recognition and Classification of Connectivity Cables
Customer
The customer is an electronics manufacturer based in Thailand that specializes in connectivity cables.
Case
Enhancing Cable Inspection Process
The customer sought a solution to improve the product quality of their USB cable inspection process, ensuring durability, functionality, and compliance with safety standards. Additionally, they needed to conduct a comprehensive inspection of external insulation materials and connectors for cracks or damage. Upon completing this process, the cables would be classified and shipped based on their respective types.
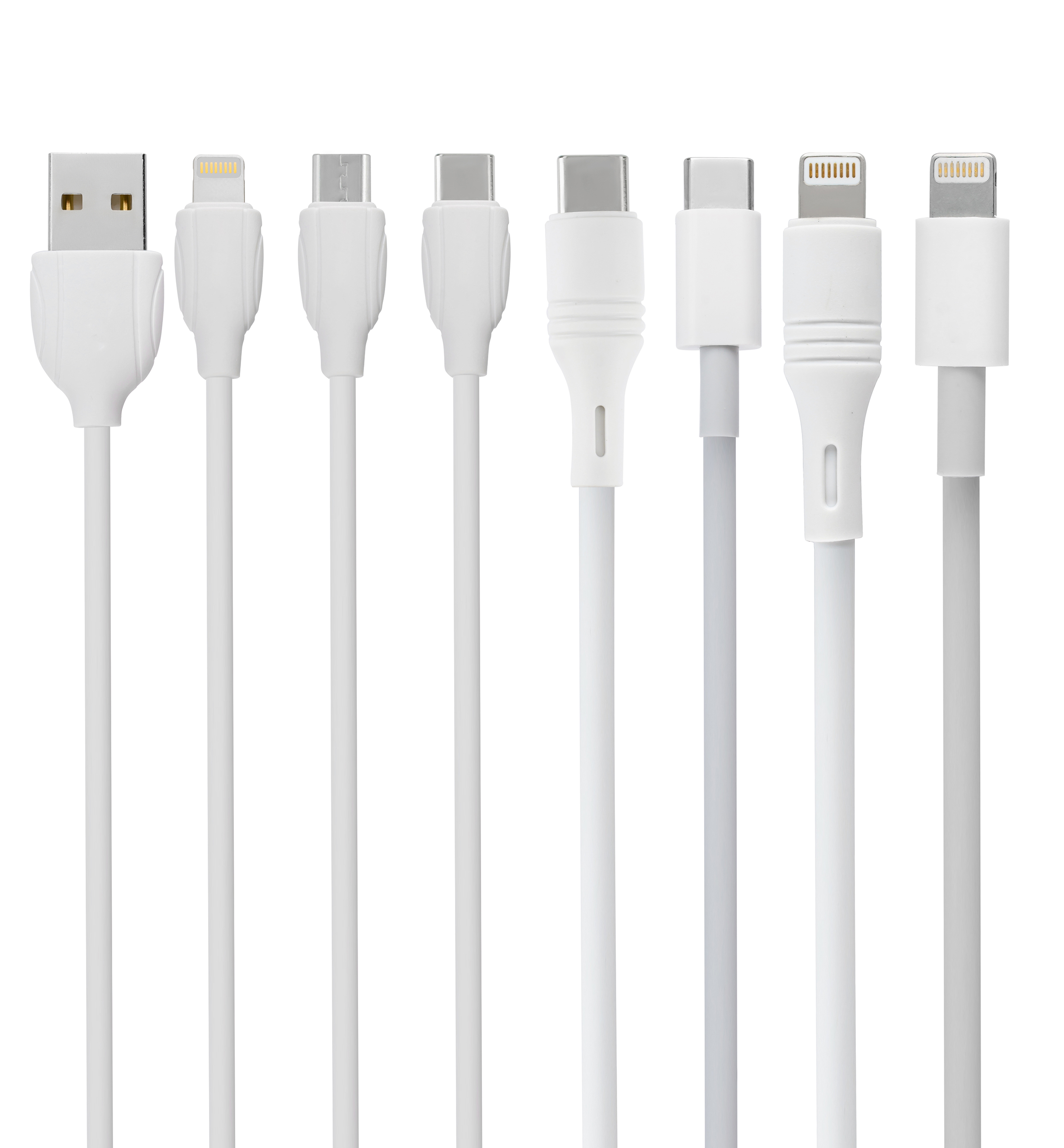
Challenge
Optimizing Quality Control and Automating Classification
Relying solely on human inspection for cables risks negligence due to fatigue, prompting the implementation of traditional AOI for defect detection. However, this often results in overly sensitive systems with strict parameter settings, leading to the erroneous identification of good products as NG (no good). This demands continuous adjustments by engineers and repeated inspections by operators.
Additionally, AOI, requiring a larger amount of samples, can only detect predefined defects, frequently missing detections (leakage) and impeding the ability to respond swiftly to flexible production demands.
Solution
AR + AI for Swift Recognition and Classification
Addressing classification, recognition, and detection challenges, META-aivi integrates artificial intelligence and augmented reality. Requiring just 5 to 10 image samples, the built-in AI quickly creates a model for swift identification and classification of cables based on type (including: Lightning, USB, RJ45, and HDMI).
Paired with smart devices, AR glasses, tablets, or portable cameras enables quick recognition and classification of different cables. The system facilitates direct transfer of related data into the company’s ERP system, generating electronic records for future reference and process enhancement. This, in turn, improves yield rates and reduces the outflow of defective products.